AI Revolution in ADHD Detection: A Psychologist's Perspective
December 17, 2024 - Reading time: 6 minutes
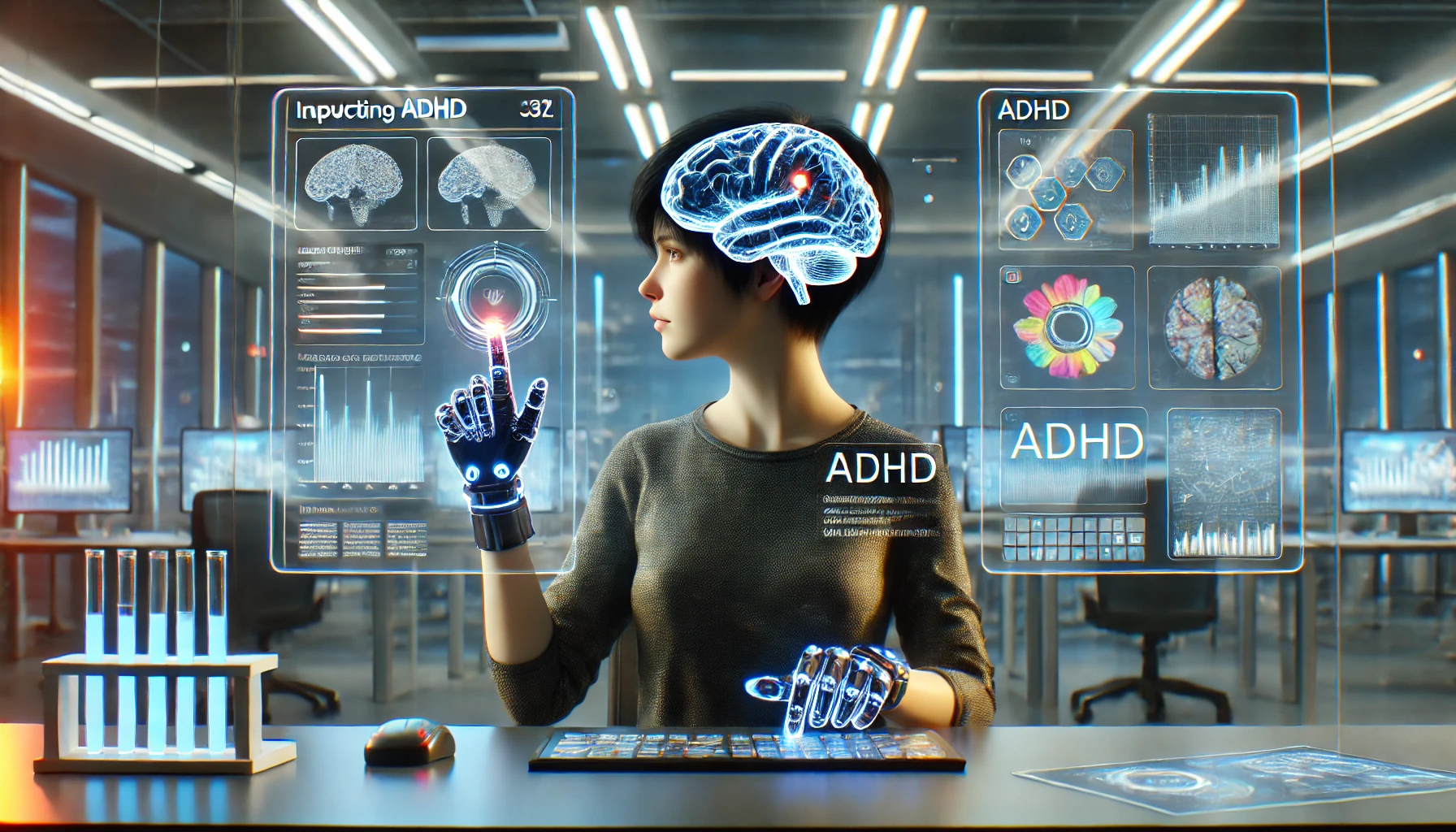
UK scientists have developed a remarkable machine learning system that can detect ADHD by analyzing video recordings of individuals performing specific tasks. As someone who has conducted hundreds of ADHD assessments, I find this development fascinating - and potentially game-changing.
The Study at a Glance
- Participants: 22 adults (ages 18-45)
- ADHD Group: 5 males, 5 females
- Control Group: 8 males, 4 females
- Test Duration: 1-1.5 hours
- System Accuracy: 95.5%
The Current State of ADHD Diagnosis
Before diving deeper into this new technology, let's consider why we need better diagnostic tools. In my practice, I've witnessed the challenges of traditional ADHD diagnosis firsthand. Take Marcus, a 32-year-old software developer who came to me last year. He'd struggled with focus issues since childhood, but because he performed well academically, his symptoms were overlooked.
Current Diagnostic Process Includes:
- Multiple clinical interviews
- Behavioral observations
- Psychological testing
- Medical examinations
- Questionnaires from family members and teachers
- Possible brain imaging studies
Beyond Traditional Testing
The Innovation in Detail
- Multiple camera angles capture the participant's behavior
- Participants complete various tasks, including interviews and reaction tests
- Small objects act as potential distractions
- The system analyzes body movements and responses
The Technology Behind the Innovation
System Analysis Components:
- Body Movement Patterns
- Fidgeting frequency
- Postural changes
- Activity levels during tasks
- Attention Indicators
- Response to distractions
- Focus maintenance
- Task engagement patterns
- Behavioral Markers
- Reaction times
- Task completion patterns
- Response to environmental stimuli
Clinical Applications and Potential Impact
Accessibility Improvements
- Reduced testing costs
- Shorter waiting times
- More widespread availability
- Easier implementation in various settings
Enhanced Diagnostic Accuracy Benefits
- More precise diagnoses
- Fewer missed cases
- Better differentiation between ADHD and other conditions
- More consistent results across different healthcare providers
Real-World Implementation Scenarios
Application Areas:
- School Settings
- Quick preliminary screenings
- Regular progress monitoring
- Early intervention opportunities
- Primary Care Offices
- Initial assessment tools
- Regular check-ups
- Treatment effectiveness monitoring
- Mental Health Clinics
- Supplementary diagnostic information
- Objective data collection
- Treatment response tracking
Future Directions and Possibilities
Key Areas of Development:
- Treatment Monitoring
- Tracking treatment effectiveness
- Adjusting interventions based on objective data
- Monitoring long-term progress
- Research Applications
- Studying ADHD patterns across populations
- Identifying new behavioral markers
- Developing more targeted treatments
- Educational Support
- Helping teachers understand student needs
- Developing more effective learning strategies
- Creating supportive classroom environments
Looking Forward
While this AI system shows immense promise, it should complement, not replace, comprehensive clinical assessment. The combination of high accuracy, multiple data points, and reduced costs could make ADHD testing more accessible to those who need it most. For the millions worldwide who struggle with attention and focus issues, this technology offers hope for faster, more accurate diagnoses and better treatment outcomes.
Final Thoughts
The future of ADHD diagnosis might look very different from the lengthy, expensive processes we know today. And for countless individuals still searching for answers about their struggles with attention and focus, that future can't come soon enough.